Our guest blogger for this post is Dr. Steve Watson, associate professor at the Faculty of Education, University of Cambridge, and a fellow at Wolfson College, Cambridge.
Learn more about Steve at the bottom of this page.
Capabilities and limitations of ChatGPT
I have been experimenting with ChatGPT in my work since its release last year. At the same time, it prompted me to reflect on how the technology works. In this blog, I want to illustrate the use of ChatGPT by outlining some of the uses I have put ChatGPT to in my teaching and research; what I have learned from thinking about the use of this technology in teaching and research practice; and finding practical ways for it to contribute to my work, as well as thinking about how the technology works as I engage with it. While I have spent a lot of time exploring ChatGPT since its release last year, I have realized that this technology had many potential applications for my teaching and research, and I wanted to realize these possibilities. However, ChatGPT is not an out-of-the-box solution. Like any technology, it takes time to learn how to use it effectively and understand its capabilities and limitations.
…one is given the impression that one is interacting with an intelligent being.
It seems to know stuff.
Like many people I have spoken to, my initial impression was that the responses that ChatGPT provides to text input or ‘prompts’ by the user appear ‘intelligent.’ As a chatbot using natural language processing (NLP) and sophisticated deep learning technology, one is given the impression that one is interacting with an intelligent being. It seems to know stuff. It is tempting to ask factual questions, thinking that it is a really sophisticated search engine or database. This is a misunderstanding, as it doesn’t hold information or look things up in the same way as a search engine or database. Deep learning, a subset of machine learning which is itself a subset of Artificial Intelligence, involves a hierarchically recursive process to identify structures in the data it is trained on. Then, when presented with new data, deep learning ‘recognizes’ its structure based on its training. ChatGPT goes further than this by identifying a structure or pattern in the prompt data and then returns the most probable next word based on the structure that it recognizes. Each new word results in a new probability for the next and so on.
Hundreds of billions of words and parameters
While this provides a simplified overview of deep learning, it makes the point that ChatGPT is not looking up or referencing information sources but is based on its training of publicly available text on the internet (around 300 billion words) with a statistical model that involves some 195 billion parameters. This is really about the probability of the next word based on recognizing the context. Within these structures, there is knowledge and information, but there is also the possibility of factual inaccuracy or misinformation. This is especially pronounced when the input prompt is open-ended, like, for instance, “what is the meaning of life?” or “how much will it cost to repair my roof?” ChatGPT is concerned with structure and form rather than factuality.
And no doubt, like many others, I began by asking fairly banal questions as if I was typing into a search engine but expecting a considered and factual response from the AI. This was disappointing because while the output text was logically, grammatically, and stylistically consistent, there were often things that were simply untrue. Having reflected on how the technology actually works, I began to work in a different way with the technology, and this is where I have had the most promising results. Not that ChatGPT really ‘creates’ anything for me; it simply assists me in developing richer interpretations through a closer and more thorough assisted reading of a text.
Use cases – automated assistive reading and writing
I get pieces of text sent to me by students and colleagues. I receive texts from prospective PhD students, other academics, and as an editor of a journal. This can be a problem in that I don’t have the time to always engage in a meaningful way with all that is sent to me. So, I began experimenting by inputting some of the anonymized texts I received into ChatGPT.
There is a limit to the amount of text that ChatGPT can deal with in a single prompt. The solution to this is to type something like “summarize the first part of the following text…” and then copy and paste around 1500 words and try to match this to a logical section from the original text. I read the summary and compared this to the actual text. I repeat the process until the paper has been summarized. Then, I ask specific questions like “Based on all the sections of the paper above, what is the main argument?” or “How is x defined?” or “How does the author use the term y?” Effectively, it allows me to thoroughly analyze the text, refine my understanding of it, and generate thoughtful feedback.
More from DEFI
I have tried different approaches to assessment and even the analysis of interview transcripts using ChatGPT. The capability to summarize is clear, but asking questions about the forms and structures of the text is possible and leads to some good results. However, this is not in a reliably automated way and requires manual checking for fidelity and plausibility. Nonetheless, this is certainly more thorough than what I could achieve manually. In this sense, ChatGPT becomes a reading assistant that helps me clarify the questions and feedback that I want to respond to the author with.
Using ChatGPT as a writing assistant
As a writing assistant, understanding the importance of prompt engineering in ChatGPT has helped me generate content in considered ways. There are two main ways in which I use ChatGPT. The first one is when I have a few ideas, and I am not sure how to structure them. I can type something like “write three paragraphs that explain/describe/argue the following…” and then list the ideas. The output from this is treated as an iteration, which provides me with a structure and form that I can then adapt and develop manually. I may also input this output again into ChatGPT to consider different structures, wordings, and phrasings. I have realized that writing iteratively, i.e., drafting and redrafting, is something I do manually. ChatGPT simply automates some of these iterations. Effectively, it can help me overcome obstacles to writing, such as writer’s block.
The second way I use it is when I am writing more fluidly, but probably not so fluently. Ideas and words are flowing, the articulation of the ideas is good, but I feel I can lose a sense of structure, or the overall argument becomes lost to me. I then input the text and ask ChatGPT to describe the argument and structure and/or the claims and assumptions that I am making. This can be very revealing, as it becomes much more apparent what I am trying to say and how the structure might be improved. In terms of the use of language, both formal and informal emergent grammar are recognized. Therefore, feedback on presentation is pragmatic rather than pedantic.
As a writing assistant, then, I feel ChatGPT deals with issues of structure and form, which frees me up to address the more nuanced and conceptual aspects of the content, knowing that there is going to be a logical organization with ‘i’s and ‘t’s dotted and crossed appropriately.
Conclusion
While ChatGPT has limitations, it has the potential to be a useful tool for teaching and research. By experimenting with different use cases, I have found practical ways for ChatGPT to contribute to my work. One of the key elements of this is recognizing the importance of the prompt so that the technology is clear about what you want it to do. This is being referred to as ‘prompt engineering’. As a writing assistant, ChatGPT deals with issues of structure and form, which frees me up to address the more nuanced and conceptual aspects of the content. While it is important to be aware of the technology’s limitations, ChatGPT can be a powerful tool in both teaching and research.
My next step is to look into developing apps based on ChatGPT that refine and automate some of the practices I have developed through this early experimentation. Such apps could help users quickly and accurately summarize large amounts of text, generate outlines or drafts for written pieces, and analyze complex interview transcripts, among other things. However, as with any technology, it is crucial to exercise caution and critically evaluate the output to ensure the accuracy and reliability of the results.
In summary, I believe that ChatGPT has the potential to be a valuable tool for researchers and educators alike, and I am excited to continue exploring its applications and potential.
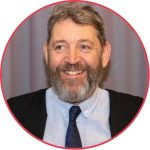
Steve Watson
Associate Professor, Faculty of Education, University of Cambridge and Fellow, Wolfson College, Cambridge
Steve Watson teaches sociology/philosophy of education, mathematics education and contributes to the training of mathematics teachers. He is currently chair of the Knowledge Power Politics research group. His research is interdisciplinary, drawing on systems theory he works across the disciplines of sociology, philosophy, psychology, mathematics, science and technology. His research themes include mathematics education; politics, education policy-making and media; professional learning; and AI in education. Before becoming an academic he worked as a secondary school mathematics teacher and prior to that as a telecommunications engineer. He is also offering workshops on the use of ChatGPT in higher education.
Thank you very much for your insights.